Stay up to dat with the future of sales
Deeper insights, better outputs: our latest AI update
We’ve equipped uman with the latest OpenAI o1 model. This new AI model enhances the quality of uman’s outputs significantly. Now, our AI agent offers you better reasoning, more nuanced details and consistent high-quality results.
In a nutshell, this is what you can expect of the new update:
- Deeper reasoning
- Better formulation
- A more detailed and nuanced approach
- Linking back to the sources
All of this is possible thanks to the new OpenAI update. The o1 model is equipped with a multi-step reasoning capability, which really sets it apart from its predecessor.
These improvements will take your sales preparation to the next level, and you’ll be able to impress your prospects and customers with detailed, resonating value propositions.
In this video, our CEO Charles compares the outputs before and after implementing the o1 model. Trust us, you'll be amazed by these improved outputs!

Sales Leader Nico Ivens (JDI) about setting the right KPIs and kickstarting your cross-sell game | The Future of Sales Leadership Podcast
In The Future of Sales Leadership Podcast, we talk about the challenges of Sales Leaders and how other seasoned Sales Leaders prepare for them.
In this week's episode, we welcome Nico Ivens. After spending over a decade working in several sales positions at ICT service provider Telindus, later acquired by Proximus, Nico noticed a successful sales strategy is built beyond the sales teams. That’s why today, he firmly believes a customer-oriented spirit should be embedded throughout the whole organization. He even wrote a book about this new way of doing business, called ‘Sales 4.0’. Since 2011, Nico has been coaching sales teams with his company JDI.
Together, we talk about why sales teams should have marketing KPIs and vice versa, why selling is more complex today and how tools actually should be embedded within your organization.
Discover the whole episode now:
.png)
Sales Leader Fabrice De Windt (Proximus) about engaging your team and the scientification of sales | The Future of Sales Leadership Podcast
In The Future of Sales Leadership Podcast, we talk about the challenges of Sales Leaders and how other seasoned Sales Leaders prepare for them.
In this week's episode, we welcome Fabrice De Windt, who leads the One Tribe segment at telecom provider Proximus. One Tribe represents a portfolio of the most strategic and complex customers Proximus services. Next to that, he’s also responsible for taking the lead in implementing AI-driven strategies and tooling within the sales chapter.
In this episode, we talk about how you should be motivating and supporting your team, why breaking a promise can cost you greatly when selling services and how sales used to be an art, but today resembles more of a science.
Discover the whole episode now:

We raised 1,9 million euros - this is what's next!
We have some great news to share! We raised 1.9 million euros to secure our international growth. The funding round is led by Holland Capital, together with existing investors imec and Seeder Fund.
We built our sales AI agent uman, to help B2B sales that operate with a broad and complex range of products and services. We know that customers’ expectations are sky-high, sales departments are facing shrinking budgets and on top of that often struggle to master that complex portfolio, resulting in lost opportunities.
We’ve designed uman to be different. It’s the first AI agent specifically built for complex B2B sales. Thanks to smart data governance and generative AI, uman can offer relevant sales content and insights at the right times. This way, sales teams are able to meet up with clients with personalised pitches and offers, without having to do all that research and preparation manually. This results in higher conversion rates, increased efficiency and more cross-sell opportunities.
Next steps
Today, several Western European leaders in IT, consultancy and telecoms are already using uman, including Proximus, msg global and the Belgian media conglomerate DPG Media, proving it works. With this investment, we want to secure our international growth. Our focus for now remains Europe, but we definitely have the ambition to conquer the US as well.
Next to that, we’ll also be focusing on product development. We have many new features planned on our roadmap, that will make uman even more performant, and plan on working on adding more integrations with different tools.
We are in the process of completely changing the way sales teams work by helping them every day to do more of what they really add value to: building relationships with their customers. This was our mission from day one, and it will remain that way.
Read more in these publications by De Tijd, Datanews and EU Startups.
.png)
Evaluating AI Assistants: Microsoft Copilot vs. specialized sales tools
Last year, Microsoft launched its own AI-powered assistant: Copilot. A real timesaver and great tool for the typical knowledge worker - but it lacks the specialized intelligence to become a staple in your sales enablement strategy. In this blogpost, we highlight the biggest differences between Microsoft’s Copilot and an in-depth AI sales assistant such as uman, and which one is the best fit for your organization.
Microsoft’s Copilot is mostly an AI add-on for Microsoft products, which provides real-time suggestions to improve documents, presentations, and spreadsheets. It’s designed to improve users’ productivity by leveraging the power of AI to reduce time spent on routine tasks, such as data entry or note taking.
Based on the demos we have seen, it looks like an engineering marvel for the local productivity tasks -which means the AI is extremely effective within said tool, but is unfortunately also siloed within that tool’s context.
That’s also the biggest difference between Copilot and a tool like uman. Most sales AI assistants will pull their data from several sources, both internal and external. We can't speak for every sales enablement tool on the market, so we'll just stick to uman for this example. Our AI fetches its data from:
- External data on the prospect, e.g. annual reports, the latest news or LinkedIn updates.
- Notes and meeting transcripts from your CRM
- Your up-to-date product information
- Your cases portfolio
- Branded templates, e.g. Word, Powerpoint, Google Slides etc.
- Sales frameworks and methodologies
- Your deal stage
Combining all those different data sources, our AI is able to generate personalized sales content that follows the sales process, brand guidelines and makes use of the right and up-to-date product information.
Garbage in, garbage out
To generate the right output, a generative AI tool needs the correct data. ‘Garbage in, garbage out’ is a frequently used saying within the AI community. How cleaner your data, how better the output. If you have little control over the data you’re inputting, you risk getting skewed results. That could also be the case with tools like Microsoft’s Copilot. Having outdated materials in your SharePoint (what company doesn’t?) is risking Copilot using that data to enrich its output, giving you incorrect sales assets.
But it doesn't have to be a problem. It’s often manageable when your organization is small and doesn’t have a huge offering. But once a company starts to grow or has an increase in complexity of their product portfolios, silos start to form. This often leads to…
… marketing having their own libraries, riddled with brand templates.
… sales processes being loosely documented in several Excel sheets or presentations.
… product information being kept in different document repositories or Excel sheets.
And that will lead to your sales content repository exploding with single use sales content. Maintaining a well-organized sales Google Drive, SharePoint, Showpad… will be a true Sisyphean task. Because…
… marketing will just keep on duplicating the same one-pagers and slide decks, in order to tweak them for every new client or lead.
… every time there’s a product update, rebrand or change, all of those assets need to be edited individually.
… sales can’t find easily what they need, so they end up tweaking their own versions, which are usually outdated, diluted and inaccurate.
Should you opt for Copilot?
The biggest issue with Copilot - or any GenAI tool for that matter - is letting it loose on stale, siloed and scattered data leads to bad outputs or even hallucinations - where the AI provides false or made-up information. But again, that doesn't have the be a problem when your organization is still small or uncomplicated.
So, when is an AI assistant such as Copilot sufficient, and when should you be looking into a more specialized tool? It depends on what your goals are, the way your organization is structured and how much budget you’re willing to pour into sales enablement tools. An AI assistant such as Microsoft Copilot can be a great timesaver for every sales team and be sufficient enough in some cases, but won’t be able to replace specialized AI sales tools.

Catch a glimpse of uman's tech stack
You’re probably wondering what funky technology stack a start-up like uman uses. We’ve seen you glare! Well, let’s open the doors and show you how managed services, and more specifically serverless technology, can truly give the engineering team of a start-up superpowers 🦾.
First, we will look at some important design choices we have put forward to design our technology. Afterwards, the major building blocks of the uman technology stack are highlighted and discussed.
Design choices for (y)our Technology Stack
The early technology design choices for a start-up can make a huge difference in the “expiration date” of the software architecture. Basically, better choices will result in lower technical debt over time and keep the engineering agility high. This is one of the rare cases in computer science where trade-offs are not specifically present, better design choices don’t generally come with more engineering workload.
Also, not unimportant, remember that design choices are contextual. The context (requirements, constraints, etc) in which you are building a piece of software drives your design choices. There is no good or bad in general, but there is better and worse within a specific context.
Managed services
In recent years, managed services have become widely available for almost all popular software products. Whether you want to host a database or run a container, most cloud providers will provide a managed service for it and do the heavy lifting — network, infrastructure, scaling, logging, etc — for you.
If you think about the output a handful engineers can achieve by leveraging todays managed services versus “do-it-yourself” (I know I am exaggerating but you get the point 😉) technology of 5–10 years ago, I would not be surprised if it is a factor in the range of 10 to 100.
To maximize the business value of our product, we leverage managed services where possible. The only exception here is when the managed service comes with a disproportional pricing.
Serverless ❤️
Serverless technology is a popular item these days, and it should be as it really is a superpower for smaller teams. It is a subcategory of managed services and basically it means that you don’t manage any servers any longer. They are abstracted away, and you pay-per-use. This is especially useful if you have high elastic workloads, but also to enable very cost-efficient environments.
To give a concrete example, at uman we synchronize data from external document management systems such as Google Drive and Sharepoint. These data crunching workloads are very elastic so it perfectly fits the serverless paradigm. We don’t have any cost when we are not synchronizing data. But when we do crunch data, we can easily scale up to hundreds of instances, without having to worry about a single server.
Our chosen Technology Stack
Google Cloud
There are a couple of global cloud providers that offer a very wide range of cloud services. Most of these cloud providers offer a similar quality of service, so most likely there is not really a bad choice here. There are hundreds, if not thousands, of articles about comparing the major cloud providers so I won’t touch that subject here. But as the title might have spoiled it, the support for serverless technology was at the top of our wish list when deciding on a cloud provider.
APIs on Cloud Run
The core of the uman platform is built out of modular stateless services, which we will refer to as ‘microservices’, that can scale independently. The backend is in essence a mesh of services where functionality is cleanly separated from each other in manageable software units.
How do we run all these services at scale? Enter Cloud Run, it allows you to run containers in a breeze, without managing a single server. No need to worry about network, scaling, SSL/TLS certificates, rolling-updates, etc. Life is easy 😎.
It is important to mention that these services are defined in an API-first manner, meaning that we first write the contract to which the service has to adhere to. This allows server and client implementations to happen in parallel, with a single source of truth on how the communication will happen.
Elasticsearch on Elastic Cloud
As we are a search product, there is no way around Elasticsearch. At first, we deployed Elasticsearch ourselves on a Kubernetes cluster. We soon noticed that this was taking a significant amount of engineering time to maintain and monitor this cluster. We decided to drop the self-managed Elasticsearch cluster for the managed service by Elastic Cloud. They offer a private Elasticsearch instance in Google Cloud for you to configure and enjoy with just a few clicks. Life is even greater now 🕺.
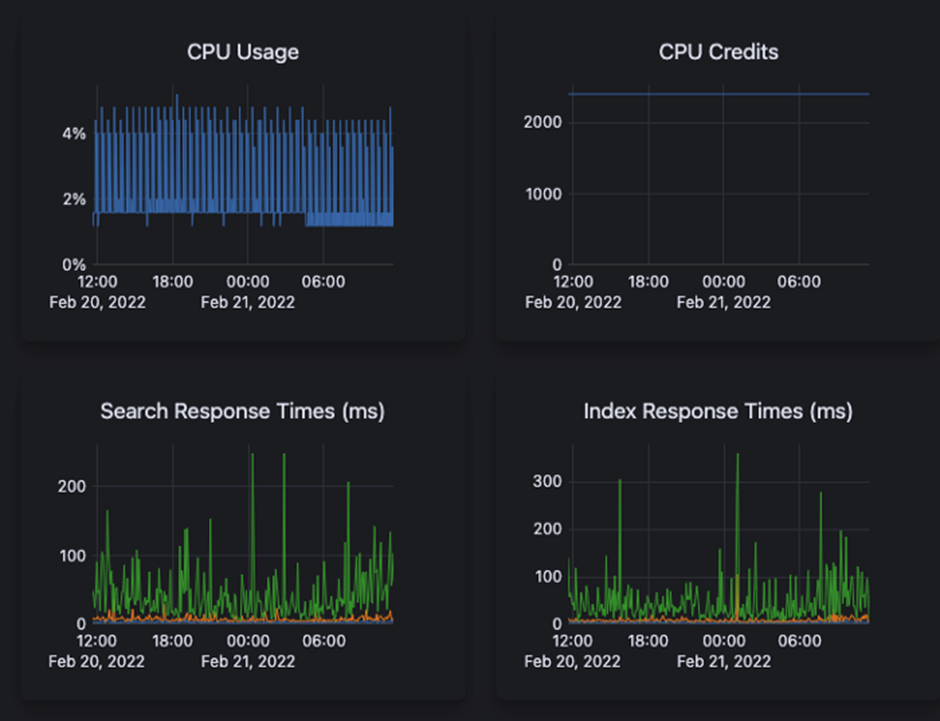
PostgreSQL on CloudSQL
To store relational data, CloudSQL is used as it offers a managed service for a PostgreSQL database. Updates, automated backups, etc is all managed for us, again with just a few clicks. This is one of the very few pieces that is not yet serverless 😩. In the future, we hope to benefit from serverless databases for our non-production environments.
Warehousing data on BigQuery
To store and transform large, mainly non-relational, analysis related data, we use the superpowers of BigQuery 🦸♂️. BigQuery is a serverless, highly scalable and cost-effective multicloud data warehouse designed for business agility.
Message queues over Pub/Sub
Messages queues have been around for quite a while now, and should not be a surprise Google has a managed, serverless service for it. We mainly use Pub/Sub to decouple parts of background processes, so that information can be processed at different speeds 🙅♂️.
(ML) Workflows on Vertex AI Pipelines
Where does all the magic happen then? Where are all the ML models trained? How do you iterate over different ML models? How do you synchronize external data with the uman platform? These are all workflows that we build and orchestrate with Vertex AI Pipelines.
Simply put, you define a set of actions that is executed in a specific order, also known as a directed acyclic graph (DAG). These actions are represented by code executions, more specifically docker containers. You can easily transfer information between steps so that every step has its necessary context to execute the right action.
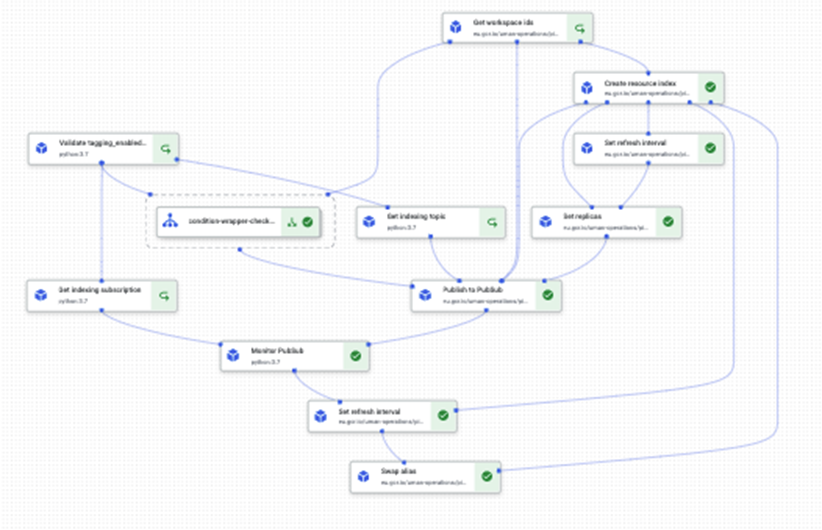
These workflows are executed in a serverless fashion, there is not a single machine you should worry about. As long as you bring your DAG definition, GCP will bring its machines and execute if for you 🧙.
API management via Cloud Endpoints
Google offers Cloud Endpoints as lightweight API management layer in order to protect and monitor the backend traffic in correspondence of the OpenAPI specification that we have set forward. This is a serverless layer that sits in front of your targeted backend service.
Unspecified API behavior (endpoints, parameters) will be blocked to avoid malicious actions to take effect. Statistics are automatically calculated for all the different endpoints and we can apply alerting on top of them. For example we want to be notified whenever search latencies start to spike 🕵️♂️.
Web app Firewall on Cloud Armor
Is this sufficient to protect your application from malicious attacks 🥷? Not really, so that is why we put a web app firewall 👨✈️ in front of the API gateway . Google Cloud Armor is leveraged to protect us from DDoS and WAF attacks at Google Scale. This includes mitigation against OWASP Top 10 risks.
Web app on Firebase
The web application, through which users connect to the uman platform, is hosted on Firebase. It offers a super smooth experience for building and running our Vue.js web application in a serverless way.

We also leverage the preview URL feature to enable a temporary frontend instance whenever a pull request is opened. In this way we can directly inspect the proposed changes 🔎.
Conclusion
As we have seen, Google offers a large suite of serverless technology that basically enables your engineering team to go 10x. And for a start-up this speed-up matters, a lot 🚀.
Are you triggered by our ingenuity? Did we leave you baffled by our experience? Are you wondering what else we have in store for you?
Never miss a thing Subscribe for more content!
See how uman can transform your sales process in a 1 to 1 demo
Discover how sales teams around the globe are using uman to streamline their sales process
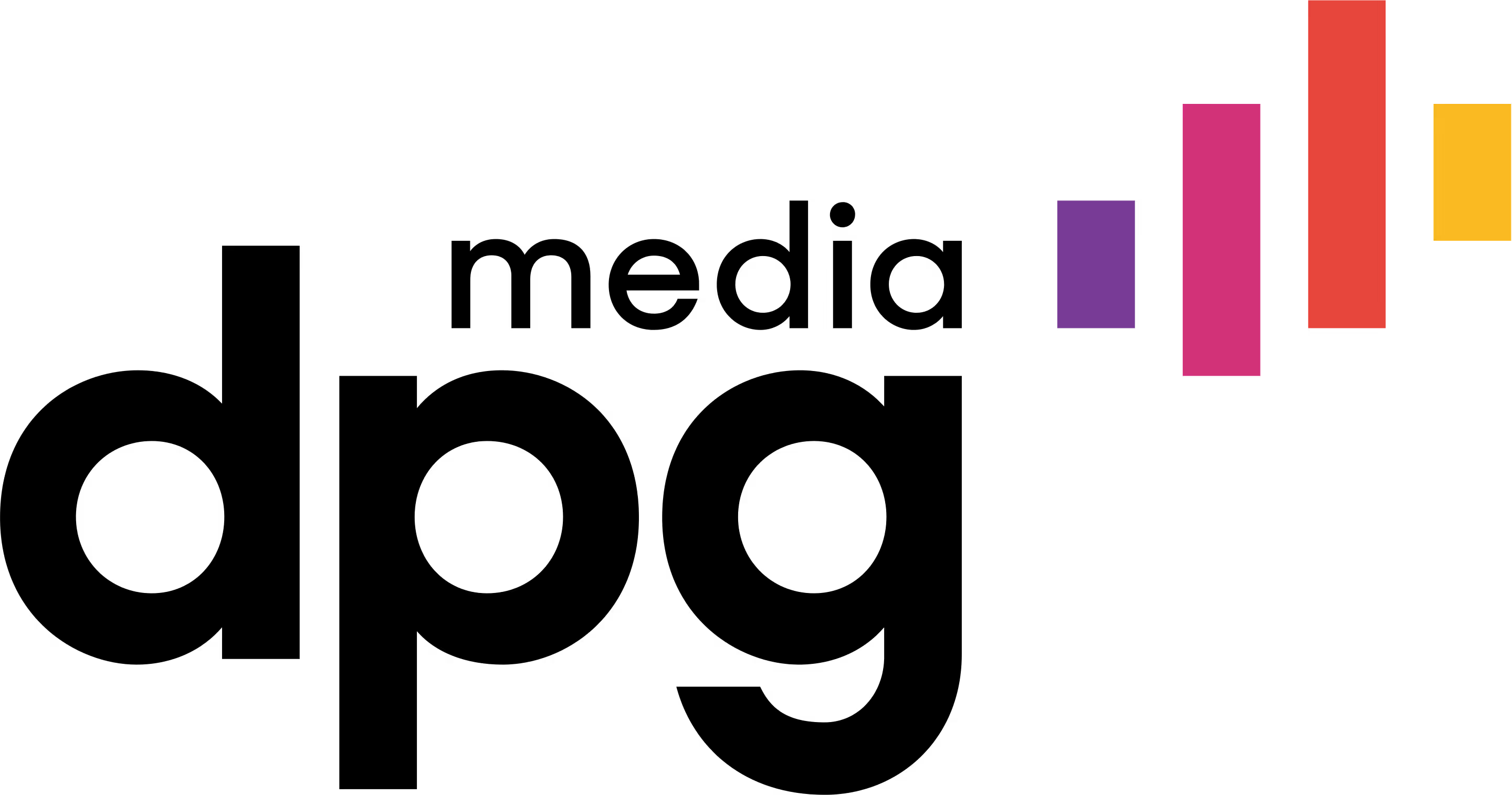
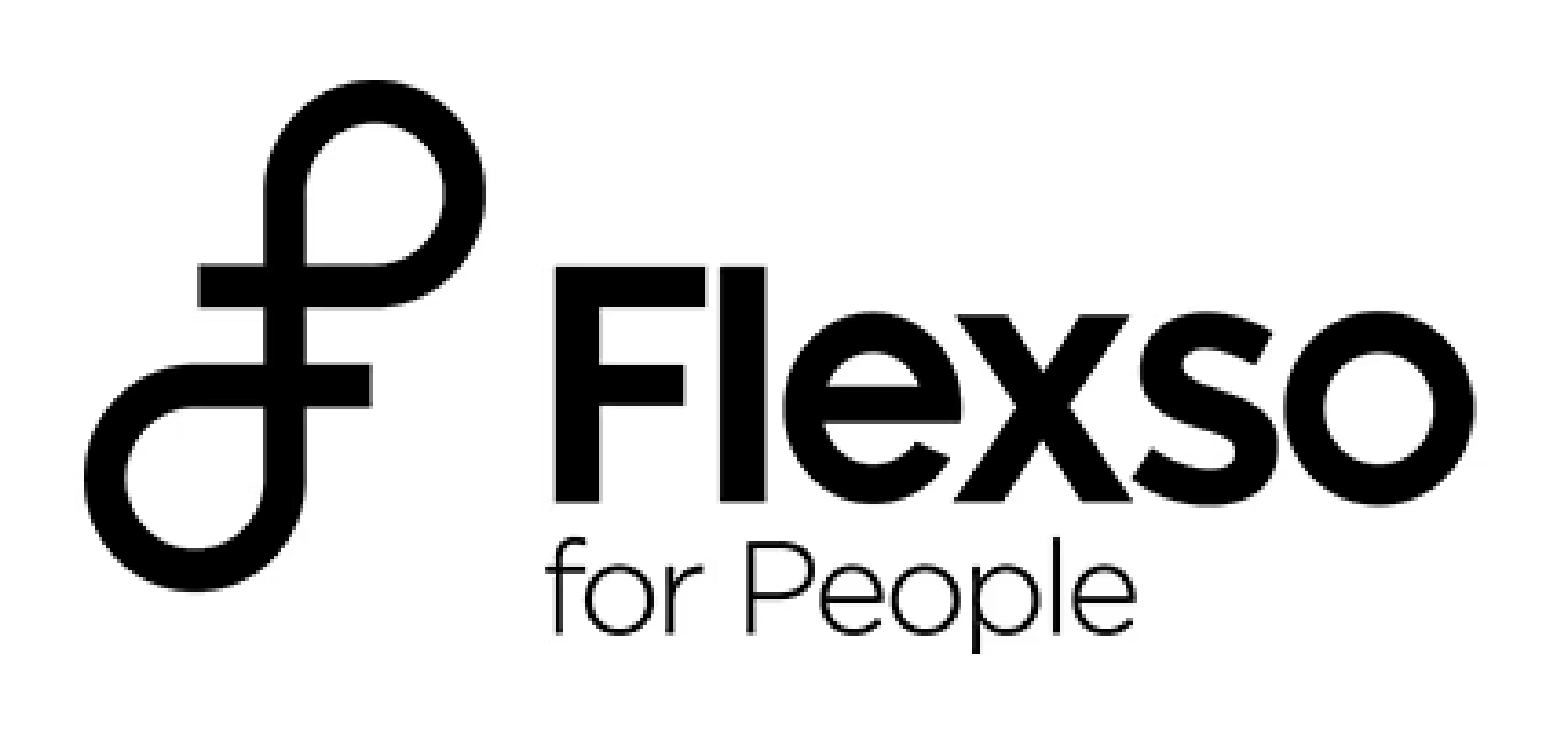

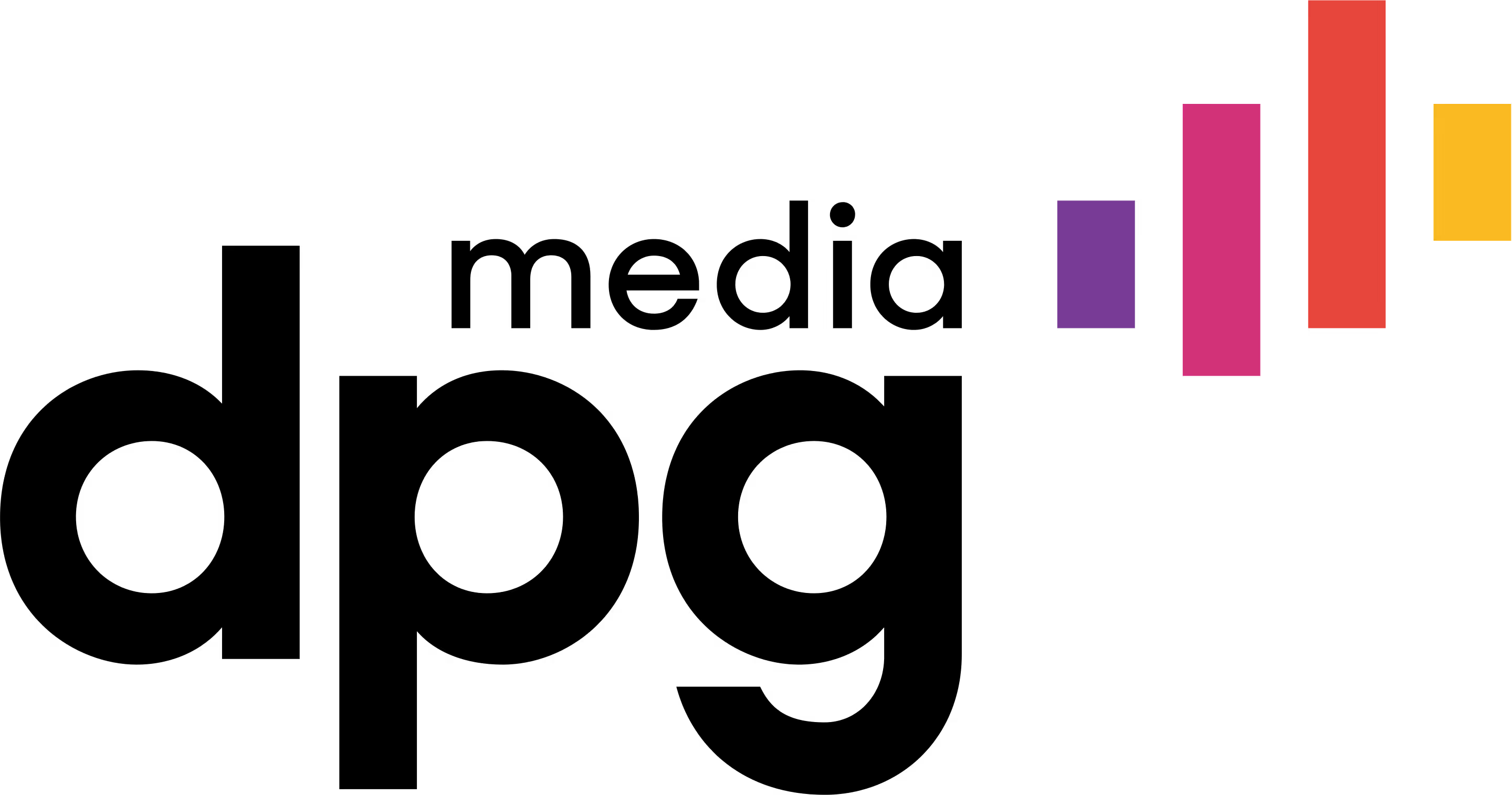
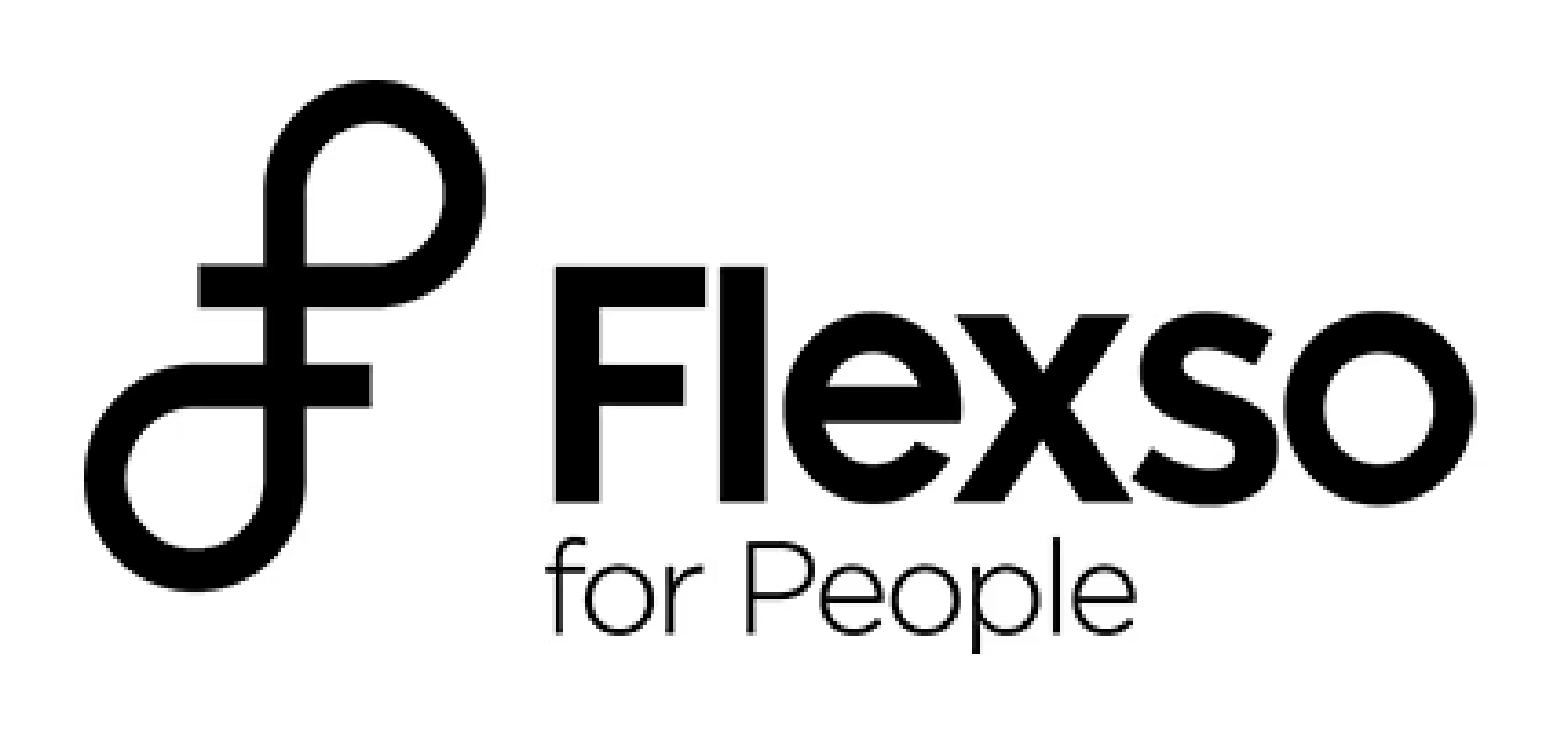

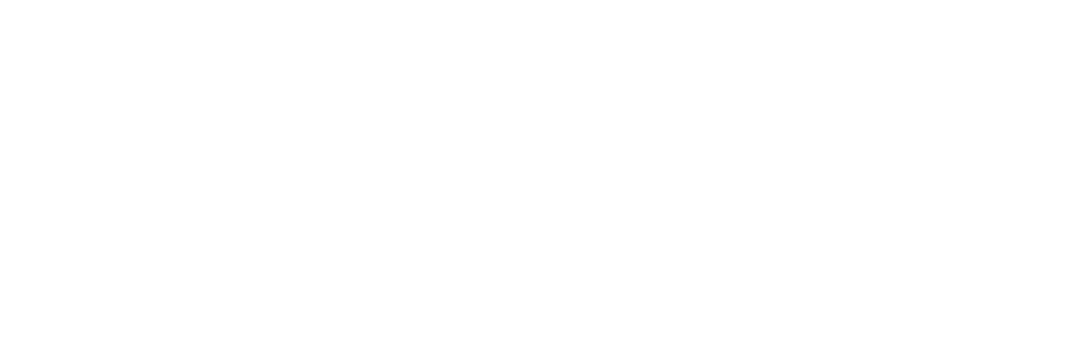
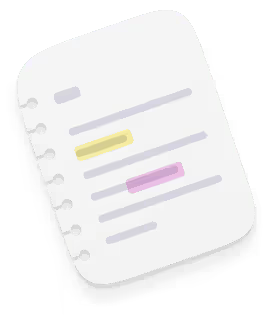
%201.avif)
%201.avif)
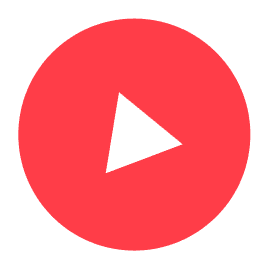